A Vision for Differentiable Control Architectures
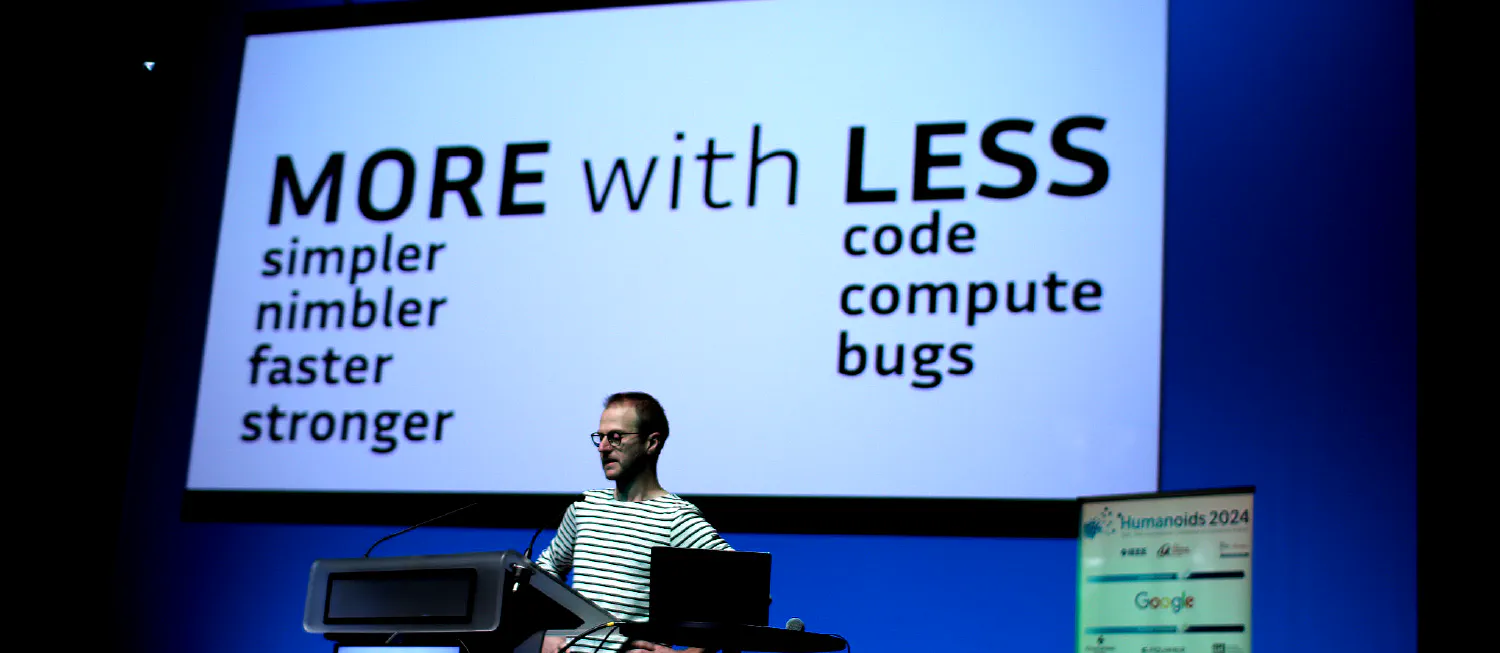
Towards Fully Differentiable Control Architecture for Robotics: Simpler, Nimbler, Faster, Stronger
Over the past decades, optimization has emerged as a key enabler for many robotics applications, particularly humanoids and quadrupeds. While initial solutions largely relied on models, in today’s data-driven landscape, it is becoming increasingly common to seek data-driven extensions to classical approaches. Differentiable control architectures propose a principled way to achieve this evolution. In this keynote, Justin Capentier did highlight recent contributions towards this objective from the team he lead at Inria, notably their efforts to (i) lay the groundwork for the next generation of differentiable simulators for robotics and (ii) develop differentiable optimization solvers that are both fast and dependable. Hopefully, these contributions will catalyze the design of the next generation of AI-driven learning and control methods in robotics.
Announcing the Maestro Consortium Initiative
At the conclusion of the keynote, Justin Carpentier publicly announced the Maestro consortium initiative. This ambitious project aims to foster collaboration among leading research institutions and industry partners, advancing the development of differentiable control architectures and AI-driven robotics technologies. Maestro sets out to accelerate progress in robotics by creating a unified platform for research, development, and real-world deployment.